—Predicts specific surface area values from sedimentation data, applied to quality control and prediction of physical properties—
Researchers) SAKAKIBARA Keita, Group Leader, NAKAYAMA Koyuru, Researcher, KUMAGAI Akio, Senior Researcher, Research Institute for Sustainable Chemistry
- Developed a high-precision model for predicting the specific surface area that reflects the shape information of nanocellulose
- Converted sedimentation behavior into heatmap images and established an analytical technique using deep learning
- Successfully predicted the properties of polypropylene/nanocellulose composites using the predicted specific surface area values
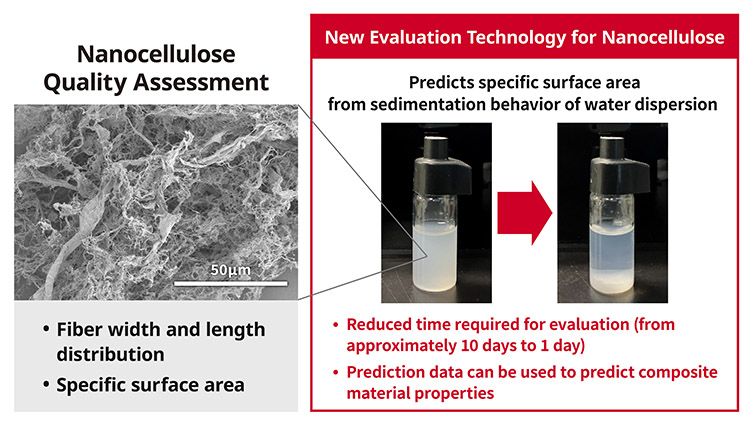
New evaluation technology based on sedimentation behavior of nanocellulose
AIST researchers have successfully developed a method to predict the specific surface area of nanocellulose from sedimentation measurements using machine learning technology. Since nanocellulose is derived from carbon-neutral biomass, it has great potential as an environmentally friendly material and is expected to contribute to the realization of a circular economy. The shape-derived properties of nanocellulose, such as fiber width, length, and specific surface area, are important parameters that determine its physical properties and quality when used as reinforcement fibers in water dispersions and composite materials. Conventional techniques used microscopic observation or gas adsorption methods for these evaluations, but the pretreatment and measurements required a great deal of time and effort (approximately 10 days). On the other hand, although the behavior of nanocellulose as it gradually settles in a water dispersion contains information on its shape, there was no effective way to extract this information. In this research, we successfully developed a prediction technique that can obtain shape-derived information easily and quickly (in about one day) by relating the sedimentation behavior and specific surface area through machine learning. This technology can be used for quality control, as it allows for easy evaluation of differences in the shape of nanocellulose. Furthermore, based on the correlation between the specific surface area and the physical properties of products using nanocellulose, this technology can be used to predict the physical properties of polypropylene/nanocellulose composite materials. As a result of the above, this technology is expected to broaden industrial application of nanocellulose throughout the industry.
Details of this technology were published online in Carbohydrate Polymer Technologies and Applications on February 4, 2025(Open Access).
Nanocellulose, a naturally occurring cellulose nanofiber obtained by fibrillating from plant biomass fibers (wood, grass, etc.), has been playing an increasingly important role in the circular economy, which aims to achieve both resource recycling and economic growth. Nanocellulose has garnered attention as a sustainable alternative to petroleum-derived resources, and is expected to make a significant contribution to promoting carbon neutrality and reducing environmental impact. In addition, its lightweight and high-strength properties have led to its use as an alternative to plastics and as a lightweight material in a wide range of fields, including automotive parts, packaging materials, and sporting goods.
However, one of the challenges in promoting social implementation of nanocellulose is the difficulty in understanding the variation in fiber morphology (fiber width, fiber length, branching, etc.) caused by differences in raw materials and fiber breakage. Since fiber shape affects the physical properties of nanocellulose, evaluation of fiber width and length using electron microscopy and atomic force microscopy, and measurement of specific surface area by gas adsorption measurement of dried material have been conducted. However, these methods are time-consuming and labor-intensive, making efficiency improvements a pressing issue. If the shape of nanocellulose can be evaluated quickly, easily, and accurately, it will be useful for quality control of nanocellulose at manufacturing and utilization sites. In addition, through on-site evaluation, it will be possible to provide nanocellulose that is tailored to market demands.
Journal: Carbohydrate Polymer Technologies and Applications
Title: Machine Learning-Assisted Sedimentation Analysis of Cellulose Nanofibers to Predict the Specific Surface Area
Authors: Koyuru Nakayama, Akio Kumagai, Keita Sakakibara
DOI: 10.1016/j.carpta.2025.100697