– Promoting development of compact AI hardware with low-power consumption and high integration –
Researchers: TSUNEGI Sumito, Researcher, TANIGUCHI Tomohiro, Senior Researcher, and YAKUSHIJI Kay, Leader, Metal Spintronics Team, Spintronics Research Center, and KUBOTA Hitoshi, Principal Research Manager of the center
- Physical reservoir computing using a nanometer-scale spintronics device that can be operated at room temperature with high integration
- Using synchronization phenomenon to suppress thermal noise and improve computational reliability
- Promoting development of compact AI hardware for IoT terminals and robots
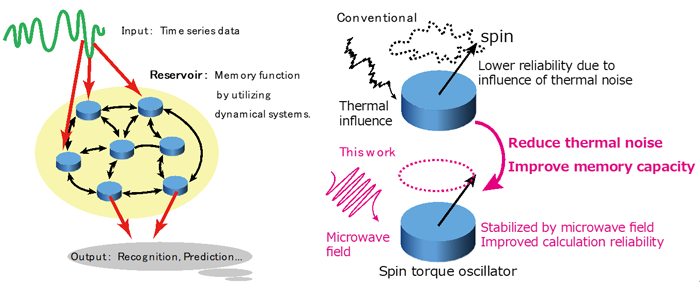
Schematic views of reservoir computing (left) and spin torque oscillator (right)
(Left) Time-series data are stored in a reservoir and computed for, such as, recognition. (Upper right) Low reliability due to thermal noise was an issue in conventional spin torque oscillators.(Lower right) This issue is solved by applying high-frequency magnetic field to stabilize the dynamics in the oscillator.
In order to confront the upcoming IoT ages, further development of information processing technology is required. AI technology, such as recurrent neural networks, has attracted much attention because of its applicability to computing of time-series data. A serious issue in such a novel computing scheme is development of a large-scale network in software. Another issue is the enormous amount of calculations for learning the training data. To solve these issues, new devices, as well as new logic circuits, have been proposed for AI hardware. Physical reservoir computing is a novel concept of recurrent neural networks for classifying and calculating time-series data. Physical reservoir computing performs the learning by optimizing weights at reservoir-to-output only, and calculates the data by using the internal dynamics in physical systems. Any physical system, such as a soft material, can be used as a reservoir for physical reservoir computing, and physical reservoir computing enables development of new computing systems. These features will solve the issues in data-processing using current AI technology.
The researchers have achieved an improvement in the short-term memory capacity of physical reservoir computing using a spin torque oscillator, in collaboration with The University of Tokyo.
Reservoir computing is a kind of recurrent neural networks featuring fast learning and has the possibility of application to artificial intelligence (AI) hardware. However, miniaturizing the computational system has been a critical issue because a small system is often affected by thermal noise, resulting in the degradation of the reliability of computation. AIST has proposed physical reservoir computing using a spin torque oscillator by taking the advantage of its small size and the capability of operation at room temperature. The proposed scheme utilizes complex dynamics in a physical system for computation, instead of building a network in software. It allows us to use a physical system as a reservoir, resulting in a low power consumption and high integration. However, a low reliability due to thermal noise was still a critical issue.
The researchers improved the short-term memory capacity, which is a figure of merit for reservoir computing, by using the synchronization phenomenon of a nanomagnet to a microwave magnetic field, which efficiently suppresses the thermal noise. A great enhancement of the short-term memory capacity indicates a high computational reliability of a spin torque oscillator. The result will promote the development of highly-integrated compact AI hardware operating at room temperature.